SOAR playbooks have done a lot of good in cybersecurity operations, especially for teams with the resources to build and maintain them. They automate routine tasks and embed the knowledge of experienced staff into workflows so that their effects are multiplied. SOAR playbooks work best when the organization has well-defined policies that make the decision tree of what to do clear-cut. For example, if the policy only allows service accounts to access certain S3 buckets, then it would make plenty of sense to build a playbook that automatically suspends IAM users who violate this policy until the access is investigated.
However, anyone who’s built SOAR playbooks knows that complexity can quickly get out of control, even if you have a nice drag-and-drop interface. For example, the screenshot below shows a community playbook that automates the simple task of submitting a suspicious file to Joe Sandbox for malware analysis:
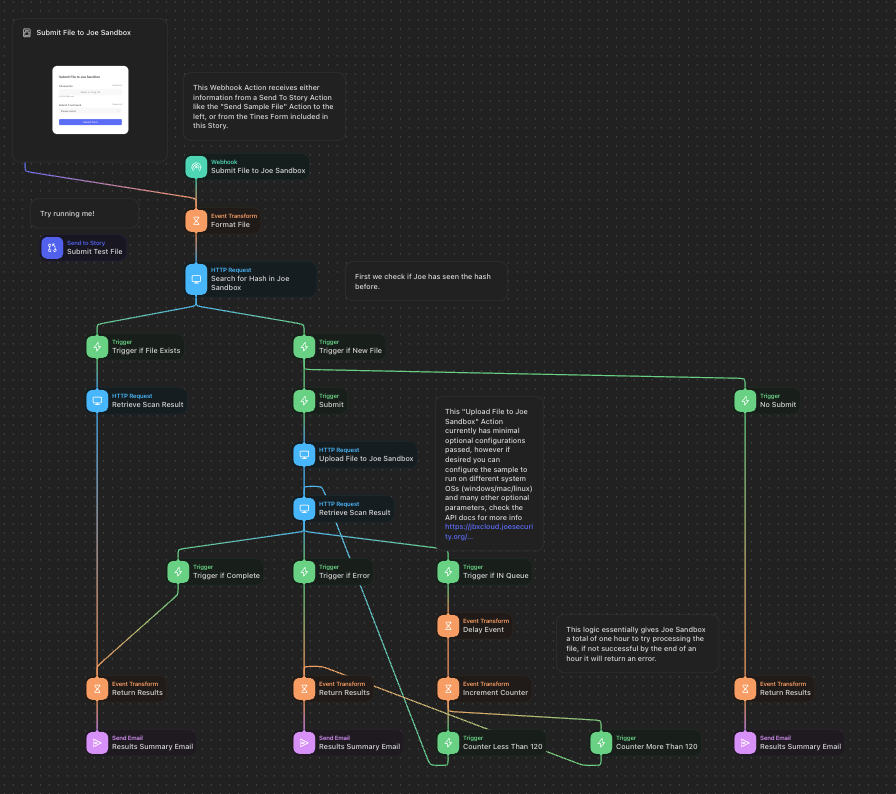
This complexity becomes costly as volume increases and because these playbooks need to be maintained. Some large security operations teams even have dedicated automation engineers who are experts at predicting what types of workflows need to be automated and then are responsible for building and maintaining the playbooks.
AI Agents Handle Complex and Long-Tail Investigations
There’s only so far that if-then logic can take your security automation. Where SOAR playbooks end, autonomous AI investigation can take over. AI agents are extremely well-suited to general investigative problems, and able to automate the investigation of many alerts that occur less frequently and wouldn’t typically have a playbook. As an example, think about the problem of autonomous driving. If-then logic might be able to route a car along a prescribed course, but AI is more suited to handle the multitude of variables you would expect driving on a real city street.
AI agents do not use if-then logic but rather recursive reasoning. That’s why Gen AI-powered automation will be able to push a cybersecurity alert investigation much further than a playbook would. Take an investigation of a typical firewall alert: An enrichment playbook will check an alert against threat intelligence and then pass it to a human analyst for the remaining 95% of the analysis. The example below is a community playbook that enriches WAF alerts with GreyNoise:
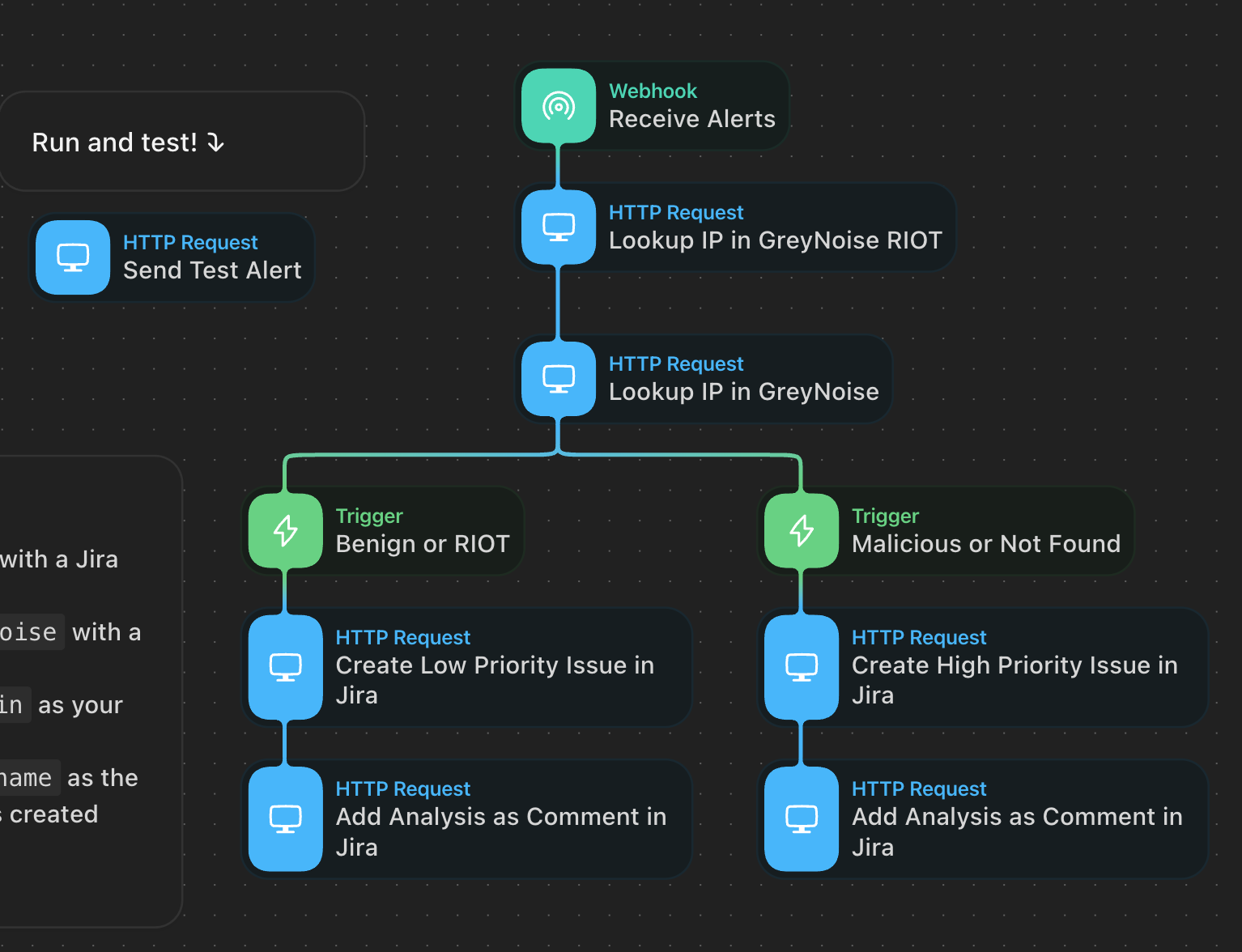
An AI agent will go much further. In the product demo below, you can see how an AI agent keeps investigating to arrive at a high-confidence conclusion. The investigation involves several recursive reasoning steps, including:
- Understanding the initial alerts from a Palo Alto Networks firewall
- Inspecting a PCAP delivered by the firewall to discover the JDNI string used in a Log4j exploit
- Retrieving firewall traffic logs to confirm the target machine connected to the malicious IP used in the exploit
- Formulating a Splunk query to pull osquery telemetry from the target machine, confirming that it’s running a vulnerable version of the Log4j software library
- Further querying osquery telemetry in Splunk to find Python3 processes using a reverse shell to communicate with the malicious IP
- Finally composing a summary report for the human analyst outlining the above, along with links to the raw data that serves as evidence

Thorough alert investigations require multiple steps that each require reasoning to evaluate the evidence, determine what the next steps are, and create the requisite queries in the right systems (firewall traffic logs, Splunk) to pull the needed data. SOAR investigation playbooks cannot automate reasoning, so they can’t pursue these types of sequential steps like an Gen AI-powered agent can.
Choosing the Best Suited Tool for Automated Investigation and Response
So the question is: Are SOAR playbooks or Gen AI better for your team? The answer is probably both and how much you use one or the other depends on your resources. We’ve built the diagram below to help you understand where investigation playbooks and Gen AI fit for different types of organizations.

The diagonal band your organization fits into above will depend on the complexity and coverage needed. Some automations are low-complexity, such as quarantining a user based on policy violation. Other automations are complex, such as figuring out whether an email is benign or malicious (see our previous blog post). Larger teams with more resources will also be able to write more playbooks to cover the long-tail of SOC workflows that occur less frequently.
Some general principles:
- Larger SOC teams with dedicated automation engineers will be able to support more playbooks while smaller teams will appreciate the assistance of AI more.
- SOAR playbooks are best suited to policy-based responses, such as immediately paging the oncall person if the data exfiltration alert is flagging one of 6 production S3 buckets.
- In situations where there are more than three variables/factors, AI-driven investigation will not only save significant playbook development time but also tend to provide better outcomes.
Saved Time Means More Cybersecurity
The premise of security orchestration, automation, and response (SOAR) is to save time. But SOAR playbooks also require an investment of time. What if your team spent 90% less time building and maintaining SOAR playbooks … what would they be able to do? It’s like asking what would firefighters do if they didn’t have to fight fires. They’d be able to do a lot! They could work on prevention and education, for example.
Gen AI promises to save SOC teams a lot of time so that they can spend more time on activities that will significantly improve security posture, such as:
- Detection engineering, creating customized alerts that Gen AI can autonomously investigate
- Table-top exercises that better prepare their organization for security incidents, including oft-neglected operational aspects such as legal ramifications and public communications
- Preventative measures to harden your environment, such as identifying storage buckets with sensitive information, creating IAM policies to enforce least-privilege access, and reducing your public-facing attack surface, such as SSH servers exposed to the internet
- Improving visibility by tuning logging mechanisms and operationalizing security tools
These are all examples of things that Gen AI cannot do, but that require the expertise of your human analysts. Request a demo of Dropzone AI to learn how you can free up your team with Gen AI augmentation.